
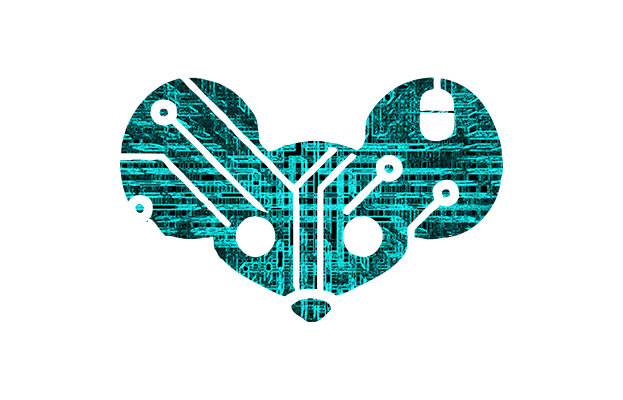
sure it does. it won’t tell you how to build a bomb or demonstrate explicit biases that have been fine tuned out of it. the problem is McDonald’s isn’t an AI company and probably is just using ChatGPT on the backend, and GPT doesn’t give a shit about bacon ice cream out of the box.
simply not true. they’re no angels or open source champions, but come on.