
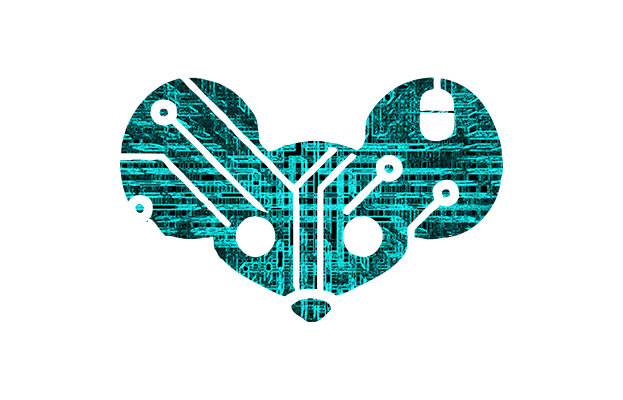
The people who are marginalised by the process are the ones who will be doing the real suffering.
The people who are marginalised by the process are the ones who will be doing the real suffering.
Hobbies indicate interest and aptitude. Someone who collects things might enjoy jobs and tasks related to organisation but not necessarily enjoy highly collaborative work that requires many meetings, whereas someone who enjoys team sports might enjoy the more collaborative social meeting type work instead of solo detailed organisation etc.
It is far from the first thing I would use as a hiring choice, but it does give me an idea of questions I might ask someone to figure out what would make them happiest.
Can we… see them?
Edit: some quick math, the minimum recommended space between trees is 3m, 200 million of those is 600km² / 148k ac , which would make the space required bigger than 17 countries (larger than Andorra but very slightly smaller than Saint Lucia). They have a blog with their tree planting updates but I wish there were more photos.
[2 mins 15 sec] Yaccarino: “I work at X, he worked at Twitter, X is a new company.”
…
[3 mins 16 sec] Yaccarino: “The team at Twitter is fantastic”
At least she lasted 1 whole minute before that embarassing contradiction. I can’t do another 38 minutes of watching that mess though, too painful right from the start.
It’s not possible to remove bias from training datasets at all. You can maybe try to measure it and attempt to influence it with your own chosen set of biases, but that’s as good as it can get for the foreseeable future. And even that requires a world of (possibly immediately unprofitable) work to implement.
Even if your dataset is “the entirety of the internet and written history”, there will always be biases towards the people privileged enough to be able to go online or publish books and talk vast quantities of shit over the past 30 years.
Having said that, this is also true for every other form of human information transfer in history. “The history is written by the victors” is an age-old problem when it comes to truth and reality.
In some ways i’m glad that LLMs are highlighting this problem.
Can’t or won’t? It’s won’t. Elon could pump a couple of million in to supplement shortfalls within a day maximum, if he wanted to.
Because nobody makes them.
Part of this is a symptom of support demands from users. There has been an expectation in software development historically, back from when software was always hideously expensive and limited to companies as users, that errors would be fixed by someone on demand ASAP. We’re all familiar with the IT guy “file a ticket first” signs on offices, or the idiot executive’s demands for a new computer because they filled theirs with malware somehow.
But now a lot of what software did is web-based and frequently free/freemium. But the customer’s expectations of having their issue fixed ASAP remains. Despite the internet being far from a standardised system of completely intercompatible components. So updates and fixes need to continually be deployed.
And that’s great for most people, until that expectation extends to the creation of new features, from management and end users alike. Then things start getting pumped out half-finished-at-best because you can just fix the MVP later, right?
We’re going to get to the backlog sometime… right? We don’t need to keep launching new features every quarter… right?
Nobody seemed to mind when the History Channel docuseries FDR came out with a British actor without a mobility disability as FDR.
Why is it that the series produced by an black female actor starring a black female actor needs such stringent accuracy controls?
Can we now start pronouncing X as ‘errrr’ when referring to the company? It would be good not to taint the good name of the letter entirely.
And it does say ‘er’ on the side of not-his-building.
it utilizes the power of attention mechanisms to weigh the relevance of input data
By applying a technique called supervised fine-tuning across modalities, Meta was able to significantly boost CM3leon’s performance at image captioning, visual QA, and text-based editing. Despite being trained on just 3 billion text tokens, CM3leon matches or exceeds the results of other models trained on up to 100 billion tokens.
That’s a very fancy way to say they deliberately focussed it on a small set of information they chose, and that they also heavily configure the implementation. Isn’t it?
This sounds like hard-wiring in bias by accident, and I look forward to seeing comparisons with other models on that…
From the Meta paper:
“The ethical implications of image data sourcing in the domain of text-to-image generation have been a topic of considerable debate. In this study, we use only licensed images from Shutterstock.
As a result, we can avoid concerns related to images ownership and attribution, without sacrificing performance.”
Oh no. That… that was the only ethical concern they considered? They didn’t even do a language accuracy comparison? Data ethics got a whole 3 sentences?
For all the self-praise in the paper about state of the art accuracy on low input, and insisting I pronounce “CM3Leon” as Chameleon (no), it would have been interesting to see how well it describes people, not streetlights and pretzels. And how it evaluates text/images generated from outside the cultural context of its pre-defined dataset.
That is not a level of power I officially possess, but it is a level of power that I am able to unofficially implement for the people who solely report to me. I am also able to tailor their roles and responsibilities to whatever causes them the least pain because their job titles are extremely non-specific, which is very helpful for both of us.
Both manager and non-managers are economically coerced into providing our time and energy. I try my best to reduce that burden for as many people as I can without being noticed by the people who are willing to suck the life out of others for personal gain.