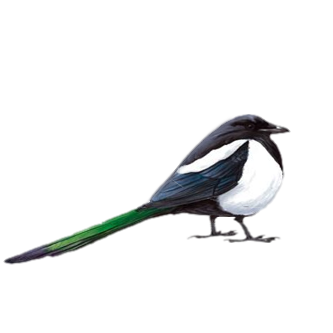
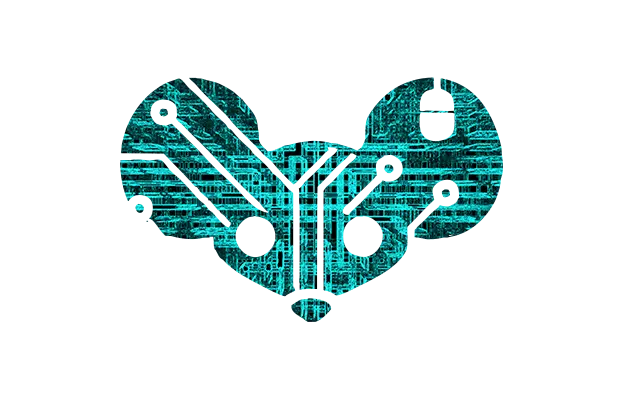
That’s not what case-by-case means. Wiktionary:
Separate and distinct from others of the same kind; treated individually.
Case-by-case implies that each treatment is different and is not generalisable; but the fact that they use a patient’s own tissue does not make each individual treatment different. If you want to extend the logic, you might call vaccination a case-by-case treatment as well, since they use different needles for each person.
I reply to people on lemmy on a case-by-case basis. I decide how to eat food on a case-by-case basis. But if you give me a deck of cards and tell me to shuffle them, I generally do not decide how to shuffle on a case-by-case basis; it doesn’t matter whose cards they are.